An Introduction to Statistical Learning with Applications in R
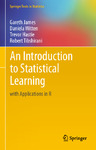
View/ Open
Date
2017Author
James, Gareth
Witten, Daniela
Hastie, Trevor
Tibshirani, Robert
Metadata
Show full item recordAbstract
Statistical learning refers to a set of tools for modeling and understanding
complex datasets. It is a recently developed area in statistics and blends
with parallel developments in computer science and, in particular, machine
learning. The field encompasses many methods such as the lasso and sparse
regression, classification and regression trees, and boosting and support
vector machines.
With the explosion of “Big Data” problems, statistical learning has become
a very hot field in many scientific areas as well as marketing, finance,
and other business disciplines. People with statistical learning skills are in
high demand.
One of the first books in this area—The Elements of Statistical Learning
(ESL) (Hastie, Tibshirani, and Friedman)—was published in 2001, with a
second edition in 2009. ESL has become a popular text not only in statistics
but also in related fields. One of the reasons for ESL’s popularity is
its relatively accessible style. But ESL is intended for individuals with advanced
training in the mathematical sciences. An Introduction to Statistical
Learning (ISL) arose from the perceived need for a broader and less technical
treatment of these topics. In this new book, we cover many of the
same topics as ESL, but we concentrate more on the applications of the
methods and less on the mathematical details. We have created labs illustrating
how to implement each of the statistical learning methods using the
popular statistical software package R. These labs provide the reader with
valuable hands-on experience.
This book is appropriate for advanced undergraduates or master’s students
in statistics or related quantitative fields or for individuals in other disciplines who wish to use statistical learning tools to analyze their data. It can be used as a textbook for a course spanning one or two semesters.